Invited Speakers
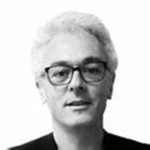
David Causeur
Institut de Recherche Mathématique de Rennes, L’Institut Agro Rennes-Angers, France
Title: Flexible handling of dependence for signal identification using functional ANOVA
Biography:
David CAUSEUR works on methods and theory in the field of statistics with applications in molecular biology and biomedical signal analysis. His main interest is the analysis of complex high throughput data such as those generated by biotechnologies used in genomic experiment. The main focus lies on the handling of stochastic dependence in large scale significance analysis and estimation of high-dimensional regression models for prediction issues. His current main research projects are in the fields of statistical learning of massive functional data for issues in epigenomics and multi-omic data integration for deciphering the cellular composition of composite samples.
David Causeur received his doctorate in statistics in 1997 from the University of Rennes. Since 1997, he was Assistant and Full Professor at L’Institut Agro, Rennes (University dedicated to biological sciences with applications in agriculture). For more than 20 years, he is a fellow of a CNRS (National Center for Scientific Research) research unit (IRMAR, Institute of Mathematical Research in Rennes). Since 2004, he is in charge of a master program in Data science for biological data in Rennes.
Abstract:
Technologies such as Near Infrared Spectroscopy (NIRS), mass spectrometry or hyperspectral imaging are now routinely used to address a variety of issues in food science. For example, NIRS is especially implemented for a rapid and non-invasive evaluation of the quality of food products. Such technologies generate high-throughput data, functional in nature and showing strong dependence across discretized observations, which optimal handling for the usual statistical tasks of testing and prediction is an open question. Originally, this research work is not motivated by issues in food science but in neuroscience, where Functional near infrared spectroscopy (fNIRS), using the absorption of near infrared light by hemoglobin to record changes in blood oxygenation, is used to measure functional brain activity. For designs in which subjects are instructed to execute a specific mental task under different experimental conditions with pre-determined levels for covariates, fNIRS provides real-time cerebral hemodynamic responses for studying neural correlates of task-related experimental variables. Data obtained from such designs are discretized observations of the hemodynamic curves on a high-resolution time scale.
The presentation will first start by questioning the gain in using statistical approaches with ad-hoc handling of dependence, with respect to “lazy” procedures ignoring dependence. In a second part, testing for overall group mean differences among curves or, more generally, relationships between curves and explanatory variables will be addressed by using functional Analysis of Variance (fANOVA) procedures in a general multivariate linear regression framework where additional assumptions are made to account for the regularity of mean curves and for the strong time-dependence across residuals.
How time dependence is modeled in such fANOVA testing procedures is crucial as it must account for the interplay between the pattern of regression parameter curves and the distribution of the time correlations. To address the challenging issue of identifying time points for which the association signal is nonzero, we propose a fANOVA testing procedure which not only flexibly handles dependence but also gives rise to optimal signal detection procedures.
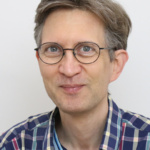
Jukka Ranta
Risk Assessment Unit, Finnish Food Authority
Title: Bayesian practices in food safety risk assessments: tricks and treats
Biography:
Since 2017, Jukka RANTA is research professor at the Risk Assessment Unit of the Finnish Food Authority, and expert on quantitative risk assessment at the unit since 2001. He is also Adjunct Professor of Biostatistics at Helsinki University, and he has been a member in various EFSA working groups and the EFSA network on Microbiological Risk Assessment, and other national or international expert groups. The cross-cutting themes have been applications of Bayesian inference & evidence synthesis in quantitative food safety risk assessments.
Abstract:
Probabilities are inherent in quantitative food safety risk assessments. This has resulted in numerous modeling applications with simulations of variability and/or uncertainty, both of which can be represented by probability distributions. Whereas variability may be described with common parametric distributions deemed appropriate for purpose, it is yet less obvious how to construct uncertainty distributions for them. Among other approaches, Bayesian methods have been applied for this over 20+ years, either for individual parameters separately, or as full Bayesian multidimensional inference. Although examples are found in research papers, applications in food authorites are less than routine. With increasing computational possibilities to simulate uncertainty and variability, there is yet very little guidance on what level of detail is needed, or whether to take a simple or more advanced approach and how. Often, Bayesian methods are placed among ‘refined methods’ in higher tiers of assessment yet-to-become, with little concrete guidance. A limiting factor is lack of resources and available tools for food safety risk assessors, since a posterior distribution is often nontrivial to solve. Yet, a cumulative toolbox of models as a library (e.g. RAKIP) and new shiny apps could provide their faster application in government food safety risk assessments. A few examples will be discussed where Bayesian methods could be ‘fit for purpose’, touching dose-response models, dietary exposure assessments, and classification, and how they could be made more accessible.
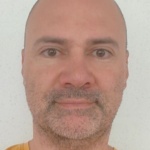
Michel Visalli
Centre des Sciences du Goût et de l’Alimentation, INRAE, France
Title : Descriptive temporal sensory evaluation with consumers: advantages and limitations
Biography:
Michel VISALLI is a research engineer at INRAE. Holder of a Master’s degree in computer science and statistics and a doctorate in food science, he designs experimental devices and develops innovative methods and software for the acquisition and analysis of data relating to food perception and consumer’s behavior. He is interested in temporal sensory measurements, indirect measurements of preferences, measurements of food supply and consumption and the use of natural language for the characterization of representations, expectations and perceptions of food
Abstract:
The transformation of food in the mouth and the release of aromatic and sapid molecules leads to temporal changes in the sensory perception of consumers. This dynamic of sensory perception has been studied for many years using temporal sensory analysis methods.
Initially, these methods were developed with trained panels, but over the last years, they shifted from quantitative to qualitative measurements, resulting in rapid methods which can be applied with consumers. While this evolution is desirable to make measurements more representative of perception of consumers, it was likely driven more by practicalities than by scientific considerations. Indeed, rapid methods compromise between costs, ecological validity and relevance of data collection.
This presentation will address different aspects related to data collection and analysis.
First, the main results of a systematic scoping review of the literature will be presented, focusing on methodological findings relating to multi-attribute temporal sensory evaluation methods.
Second, the granularity of temporal sensory data will be questioned through the presentation of the results of several studies based on data collected using a new method collecting data retrospectively to the tasting and summarizing perception in three periods
Third, the performances (validity, reliability, temporal resolution and discrimination ability) of the temporal methods will be compared using data collected on controlled temporal stimuli delivered in the mouth using a gustometer.
Finally, recommendations will be made to help practitioners choose the most appropriate rapid method of collecting temporal data based on their problem, to implement it effectively with consumers, and finally to analyze the temporal data in such a way as to be able to draw robust conclusions.
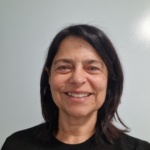
Maria Teresa Godinho
Departamento de Matemática e Ciências Físicas (IPBeja)
Title: Remote sensing data in the definition of management zones in precision agriculture
Biography:
Maria Teresa GODINHO received the PhD degree in Operations Research from the University of Lisbon. She has been with the Department of Mathematical and Physical Sciences at the Instituto Politecnico de Beja, Portugal, since 2001, where she holds a position as an Adjunct Professor. Her primary areas of interest are integer programming and optimization algorithms with applications in real problems, including precision agriculture.
Abstract:
Specific-site management (SSM) is the management of agricultural crops at a spatial scale smaller than that of the whole field. SSM techniques are relatively new in agriculture – its implementation, in the 1980’s, begun as a response to the need to control costs in large scale mechanized agriculture. Since then, its focus has evolved and now SSM techniques are also recognized and recommended for their contribution to a sustainable agricultural that does not marginalize profit. Specific-site management methods require withinfield spatial variability to be identified and measured. The problem of identifying and delineating areas with different properties is designated as the management zone delineation problem (MZsP).
This talk addresses precisely the MZsP. Namely, we show how to use remote sensing data to delineate management zones through triclustering techniques. In our algorithm, these techniques are applied to mine spatio- temporal clusters from time series of remote sensing data. Then, each cluster represents a behaviour pattern for a particular subset of spatio coordinates through a specific set of times. The temporal component of this analysis permits MZs dynamics to be characterized within timeframes in the growing season.
Data from maize and vineyard crops in Alentejo have been used to assess the suitability and accuracy of the algorithm with good results.
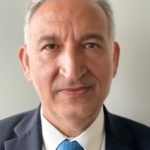
Moez Sanaa
Head of Unit, Standards and Scientific Advice on Food and Nutrition WHO HQ – HEP/ NFS Department
Title: The present and the future of food safety risk assessment
Biography:
Since 2021, Dr. Moez SANAA is head of the World Health Organization’s Department of Nutrition and Food Safety’s Scientific Standards and Advice on Food and Nutrition (SSA) unit. He was previously the Head of the Food Risk Assessment Unit at the French Agency for Food, Environmental, and Occupational Health Safety (ANSES), where he oversaw work on the risk assessment of chemical and microbiological contaminants, as well as the safety assessment of enzymes, processing aids, food contact materials, and genetically modified organisms (GMOs). He was previously professor at the National Veterinary College, Alfort, France, where he taught biostatistics, epidemiology, and risk analysis, as well as supervising national and European research programs in the field of veterinary public health (now known as One Health).
Between 2005 and 2018, he contributed significantly to the work of the European Food Safety Authority (EFSA) expert groups on animal health and welfare and biological risks in food, as well as participating in several capacity-building projects for food safety systems in Africa, Eastern Europe, and the Middle East. He served as a senior epidemiologist at the US-Food and Drug Administration’s Center for Food Safety and Applied Nutrition (FDA-CFSAN, Maryland, USA) from 2017 to 2018.
Abstract:
The future of risk assessment lies at the intersection of scientific innovation, ethical imperatives, and societal expectations. As we negotiate a fast-changing landscape impacted by advances in technology and environmental problems, conventional paradigms are being reinvented to suit the complexity of the modern world. In this period of profound shifts, a novel frontier arises, marked by the New Approach Methodologies (NAMs) based on in silico and in vitro approaches, mechanistic information (e.g. adverse outcome pathways, impact of regulated products), artificial intelligence, and omics/bioinformatic approaches. This change marks the transition from resource-intensive, animal-based models to more integrated, efficient, and mechanistically driven evaluations based on interdisciplinary collaboration.
Considering the increasing complexity of food safety issues, implementing integrated methods is critical. Through a ‘One Health’ perspective, we address chemical and microbiological hazards, as well as the emerging problem of antimicrobial resistance, while taking social and environmental concerns into account. This comprehensive strategy strengthens our defenses against complex problems, ensuring the resilience and sustainability of our food systems and the health of future generations.